Unsupervised Anomaly Detection for Medical Imaging (f/m/x)
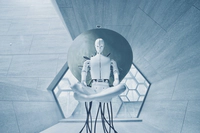
Abstract:
Unsupervised anomaly detection methods aim to detect irregular and rare data instances that deviate from the normal, expected distribution.
Although much work has been done on the use of auto-encoders (AE) to detect anomalies, it has been shown that learning the healthy distribution is stil cumbersome, with AEs being able to reconstruct some types of anomalies even better than samples from the trained distribution. Strategies to constrain the latent manifold of AE include adversarial training, probabilistic modeling, or deformable auto-encoders. However, synthesizing healthy reconstructions from pathological input scans still remains a challenging task.
The objective of this project is to develop novel unsupervised anomaly detection methods to detect and segment pathology on challenging medical imaging datasets.