Unsupervised Anomaly Detection in Medical Imaging
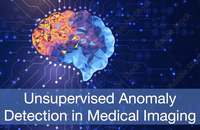
Anomaly detection aims to identify patterns that do not conform to the expected normal distribution. Despite its importance for clinical applications, the detection of outliers is still a very challenging task due to the rarity, unknownness, diversity, and heterogeneity of anomalies. Basic problem formulations to recent advances in the field will be discussed.
This includes, but is not limited to:
- Reconstruction-based anomaly segmentation
- Probabilistic models, i.e., anomaly likelihood estimation
- Generative models
- Self-supervised-, contrastive methods
- Unsupervised methods
- Clinical Applications
Please register via the TUM matching system: https://matching.in.tum.de
Check the intro slides here: